Welcome to Machine learning to substitute LLDPE plastic film with Kraft paper in automatic pallet wrapping
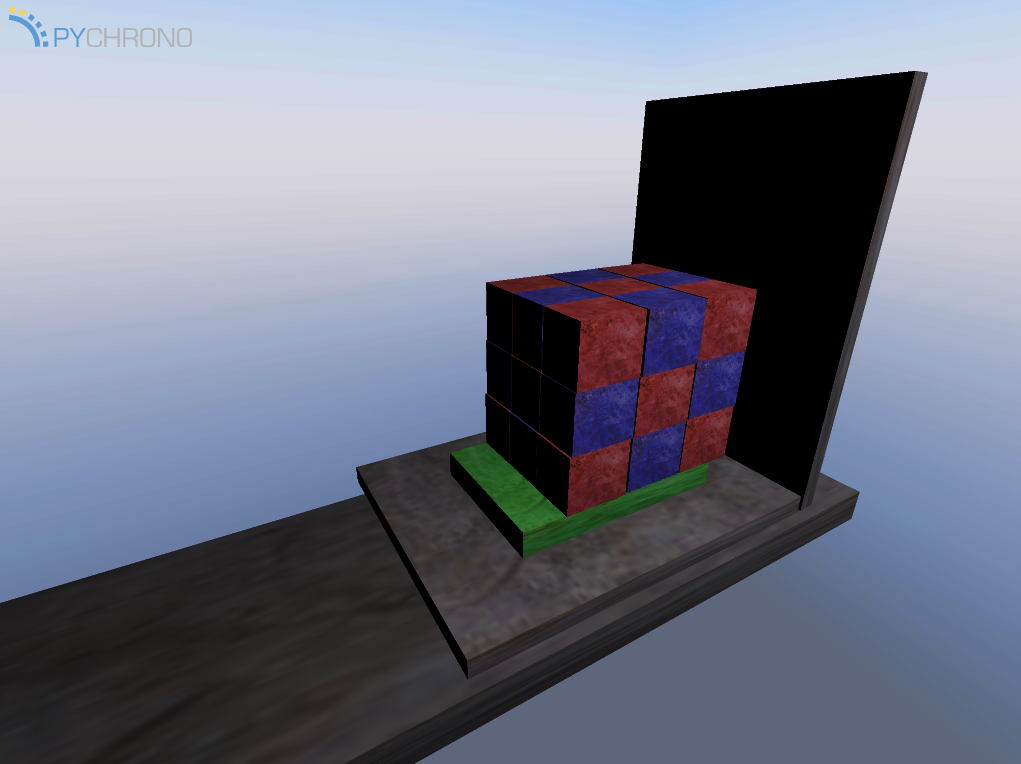
This is a project supported by ACMI S.p.A. and funded with D.M. 10.08.2021 n.1062 on FSE REACT-EU, by Ministero dell’Università e della Ricerca (MUR), under the Programma Operativo Nazionale (PON) “Ricerca e Innovazione” 2014-2020, Azione Green.
Objective: Study and model the dynamics of a loaded unit to provide intelligent suggestions for the development of wrapping formats.
Partner: ACMI S.p.A. (https://www.acmispa.it/en/) is an Italian manufacturer of high-tech bottling and packaging lines, specialized for beverages and food. ACMI has international relevance, serving both national companies and large multinational groups. The recent work of ACMI is significant in the open discussion about plastic, since their novel green approach to the end-of-line proposed to replace the external wrapping material from LLDPE plastic film to Kraft paper (a recyclable and biodegradable paper with specific elastic strength). This represents the first attempt in substituting plastic tertiary packaging for food and beverages industry. A completely plastic-free end-of-line opens up to a series of engineering and automation challenges which have yet to be explored.
Relevant pubblications:
Paper “Substitute plastic film with Kraft paper in automatic pallet wrapping: an AI pipeline” @ AIxIA 2022
Abstract
This paper presents and discuss an overview of an AI pipeline to analyze the effects of substituting plastic film with Kraft paper in the tertiary packaging, i.e., in the external envelope of a pallet. Since there is no prior knowledge about paper wrapping yet, the goal is to understand the physics of the load unit—wrapped in paper—when subject to horizontal accelerations. This permits to study and analyze its rigidity and robustness to permanent deformations and/or excessive shifting during road or rail freight, to avoid damages and ripping of the envelope.The idea behind our AI pipeline is to virtually simulate such a situation, to precisely identify critical use cases, and eventually suggest a correction in the wrapping format. The first gain in using such an approach is to drastically reduce the number of physical tests needed to build a solid base knowledge about the behavior of Kraft paper enveloping the pallet during motion. The proposed pipeline consists of three phases: (i) data collection from real tests, (ii) modeling of the simulation, fitting relevant parameters between the actual test and the simulated one, and (iii) performing of virtual experiments on different settings, to suggest the best format. Computer vision and machine learning techniques are employed to accomplish these tasks, and preliminary results show encouraging performances of the proposed idea.
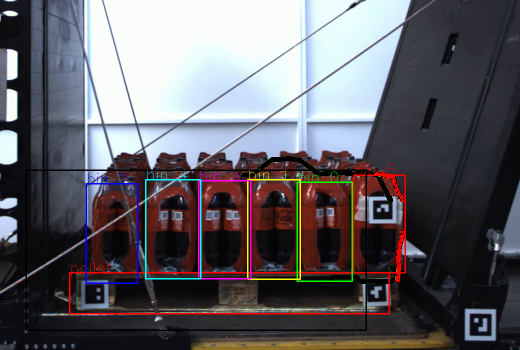
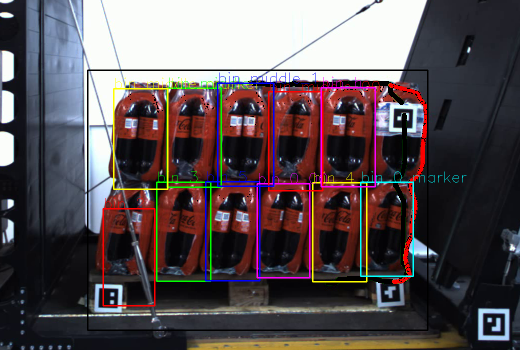
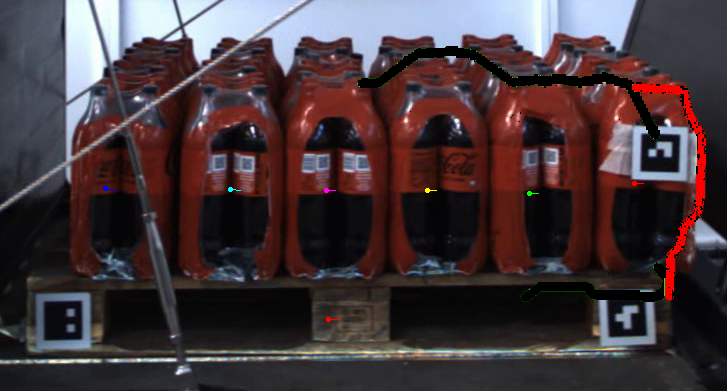
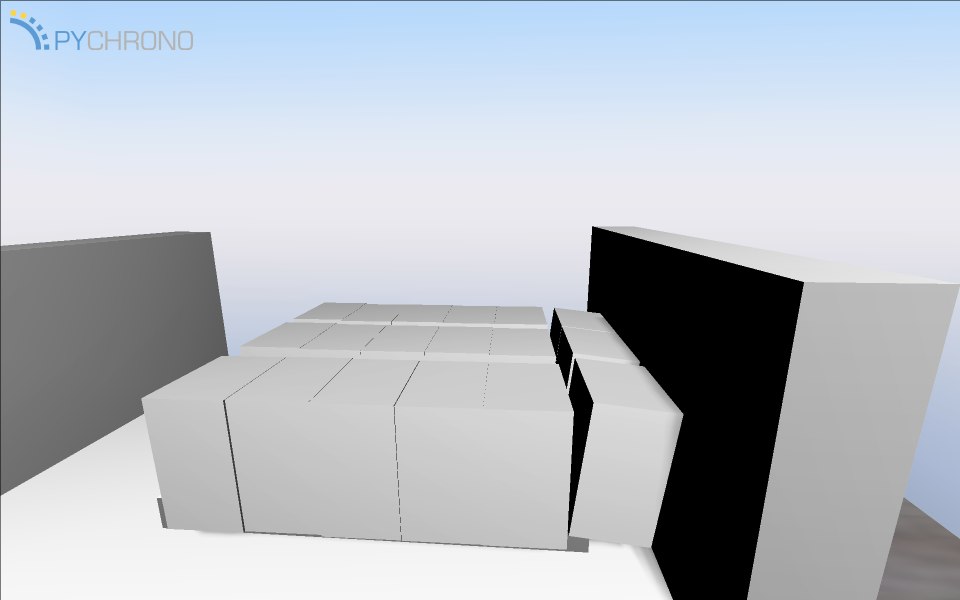
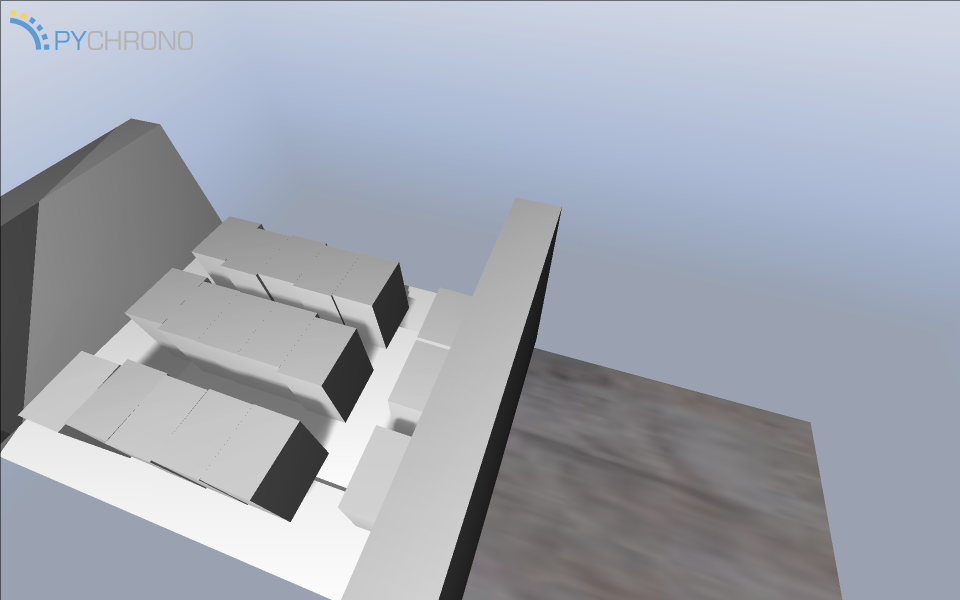