Welcome to CARING: CT-scan Automated REasoning INterpretation Guidance
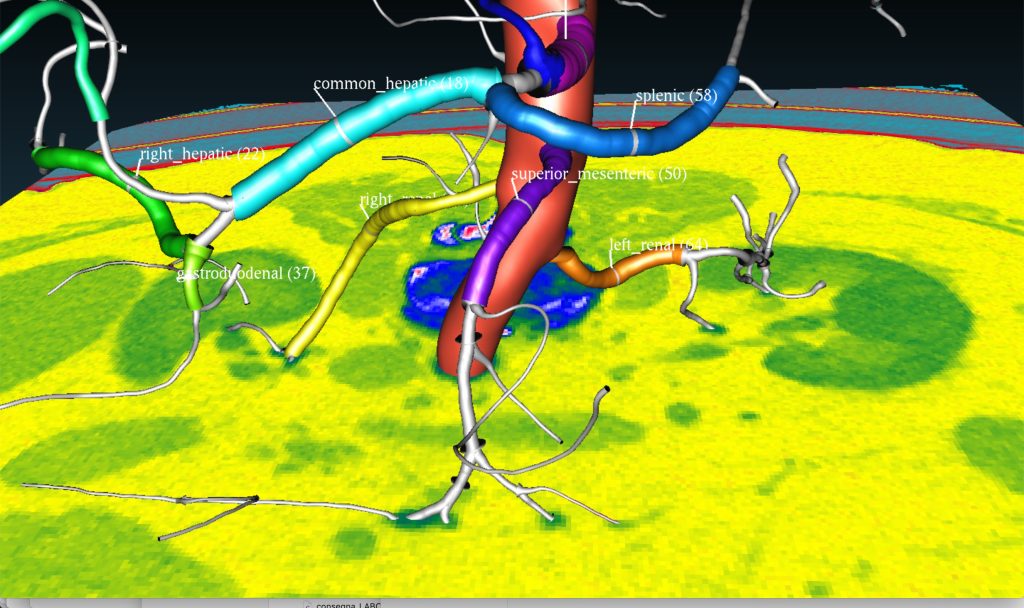
Objective: Modeling medical knowledge to deliver explainable AI interpretations of CT scans.
Brief Description: Artificial Intelligence-based segmentation of Computed Tomography (CT) scans is the first step of the automated pipeline for the identification of potential pathologies in human organs. To this end, this project studies a Declarative Programming methodology for CT scan automated interpretation. The use of a logic-based approach guarantees several advantages with respect to other existing methods which are mainly based on Machine Learning. These ML approaches are, generally, outperformed by medical doctors, in terms of precision, and require high volumes of sensitive data for training. On the other hand, our tool, based on Answer Set Programming, returns reliable, easy-to-program, and explainable interpretations that are solely dependent on the knowledge injected by the medical doctors themselves. This allows CARING to encapsulate anatomical knowledge and the experts’ experience in a reasoning process that is understandable by the medical doctors and that can be easily enriched and/or modified.
News: a new methodology for surfaces reconstruction is being developed by our lab! More info coming soon
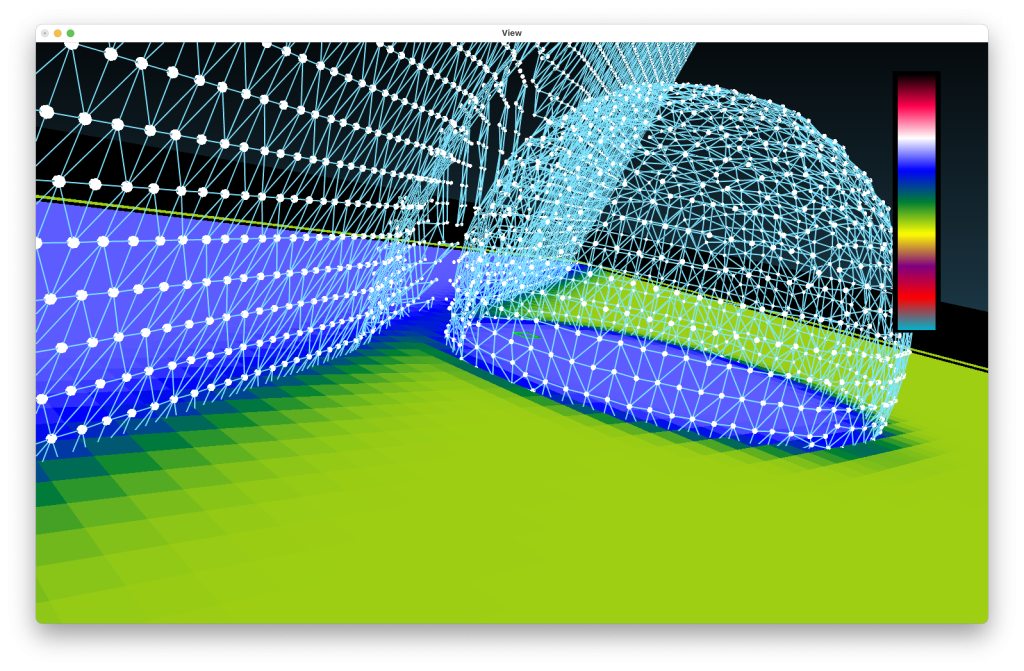
Relevant Publications:
- F. Fabiano and A. Dal Palù. An ASP approach for arteries classification in CT scans. Journal of Logic and Computation, 2022
- F. Bertini, A. Dal Palù, F. Fabiano and E. Iotti CARING for xAI. Proceedings of CILC 2022, 2022.
- F. Fabiano and A. Dal Palù. An ASP approach for arteries classification in ct-scans. Proceedings of CILC 2020, CEUR volume 2710, pages 312–326, 2020. Best practical impact paper award